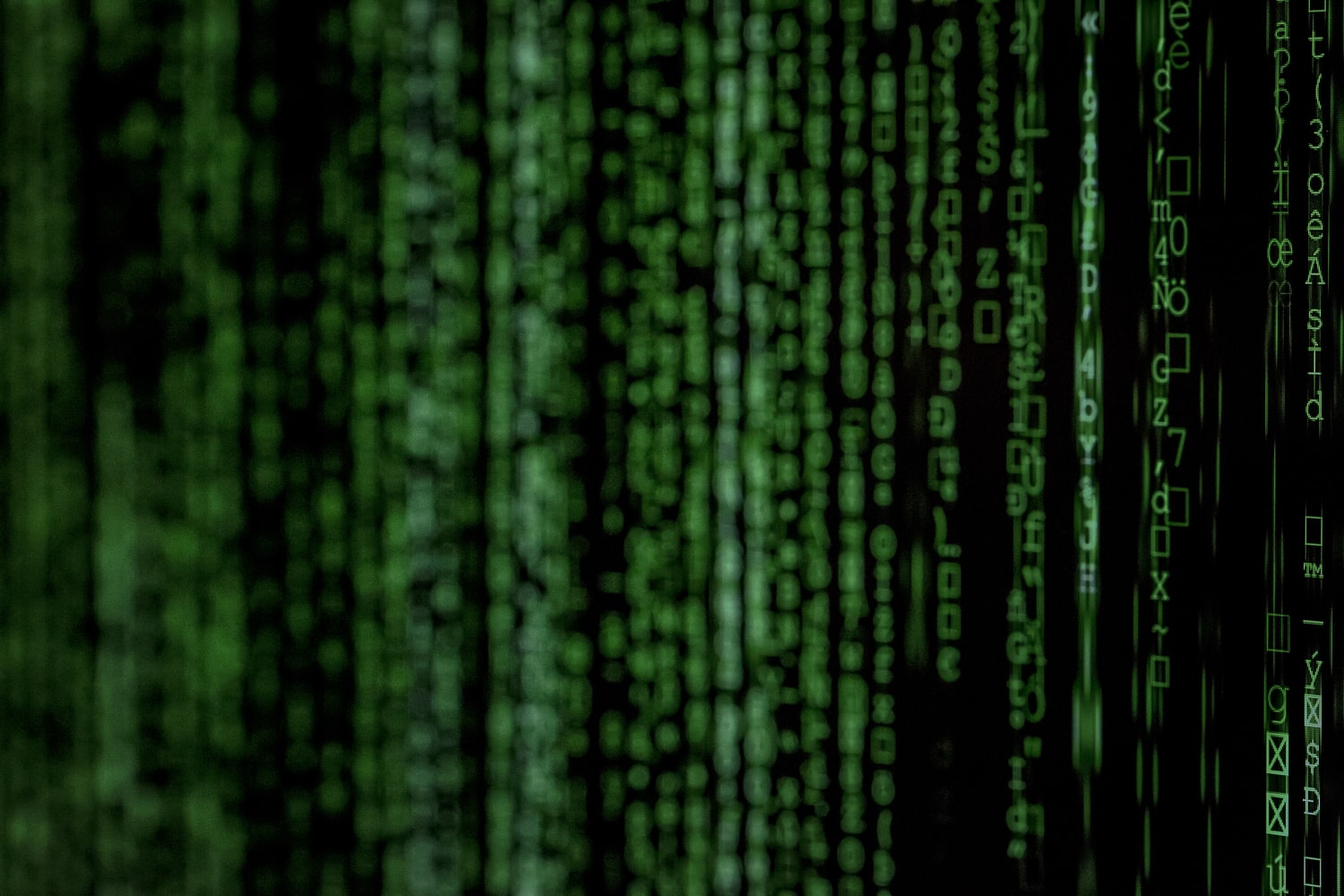
An AI test for Europe
03/11/2021New regulations for algorithmic decision systems?
Lawyer Ferdinand Müller (Research Group "Shifts in Norm Setting") and Computer Scientist Martin Schüßler (Research Group "Criticality of AI-Based Systems") have worked in an interdisciplinary team together with Elsa Kirchner, a biologist and computer scientist from the German Research Intelligence (DFKI), to develop a proposal for assessing the risks of algorithmic decision systems.
Artificial intelligence, in the form of fully or partially automated algorithmic decision systems, is being used in more and more areas of application. Such systems are used, for example, for SCHUFA credit checks, for high-frequency trading on the stock exchange, for the pre-selection of letters of application in human resources management, for self-driving vehicles or for the evaluation of medical image data in areas such as prenatal medicine or cancer detection.
A special feature of algorithmic decision systems (ADS) is that they can process extremely large amounts of data in a relatively short time because they are decoupled from human input. Under certain circumstances, these systems rely on very complex models, which makes it difficult or even impossible to reconstruct the results afterwards.
Many states are currently considering creating new laws for ADS. At EU level, the European Commission presented the White Paper on Artificial Intelligence in mid-February 2020, incorporating previous recommendations such as the expert opinion of the High Level Expert Group on Artificial Intelligence. However, the White Paper is still far from providing a solution in the form of concrete regulation.
-
© Esra Eres[Translate to English:] Interdisziplinäres Projektteam: (von links) Ferdinand Müller, Martin Schüßler und Elsa Kirchner
-
© DFKI GmbH/Felix Amsel
-
© Esra Eres
Interdisciplinary project team: (from left to right) Ferdinand Müller, Martin Schüßler and Elsa Kirchner
What unites all the expert opinions and strategies for regulating ADS presented so far is the pursuit of a structured criticality or risk assessment. A model that was presented by the Data Ethics Commission set up by the German federal government in its report published in 2019 has attracted a lot of attention. The model is based on a classical risk assessment. On the one hand, it considers the severity of the possible damage that a specific technology can cause, and on the other hand, the probability of the damage occurring. Taking into account the two factors of severity and probability of occurrence, the overall result is the classification of a technology on a risk ladder or pyramid. Depending on the classification, regulatory follow-up may be necessary. This follow-up could, for example, take the form of a specific authorisation procedure (for applications with a significant potential for harm) or self-regulation obligations (for applications with a lower potential for harm).
As an alternative to this classical risk assessment, we propose a modified procedure which, in our opinion, better reflects the specificities of ADS. Because ADS can be applied in a variety of areas and in many forms, this makes it difficult to classify them uniflormly on a single scale. We therefore believe that a matrix is more suitable for risk assessment than a one-step pyramid. The matrix model, which we propose as an alternative, focuses on the qualitative assessment of risks. At the same time, the matrix should enable its users to identify concrete measures for action.
Instead of assessing the “severity” and “probability of occurrence”, the matrix considers, on the one hand, “system-related risks” resulting from the ADS technology in question and on the other hand “application-related risks” resulting from the specific use of the technology.
System-related risks are those caused by the algorithm, model or training data on which an ADS system is based. This can lead to systematic distortions of the result (also known as “biased AI”), which can emerge due to an incomplete or short- sighted selection of decision-relevant parameters. Another problem is the lack of transparency in some ADS systems, the results of which are difficult or impossible for humans to understand and thus correct. In addition, learning ADS systems have a higher risk potential than those systems that do not change during use. Application-related risks, on the other hand, result from the specific field of application.
The use of ADS to predict the probability of recidivism among criminals affects other legal rights than the use of ADS in high- frequency trading on the stock exchange or in the evaluation of medical image data.
Two examples illustrate how the matrix works:
Example D:
A dating app is not transparent due to the large number of parameters and decision-making levels used, i.e. its results are difficult or impossible for people to interpret. This represents a high system- related risk. At the same time, the application-related risk is relatively low. Users of a dating app do not have to fear physical or financial damage. They have voluntarily decided to use the app as part of a contractual relationship. Despite the high system-related risk, such a dating app is therefore located in the green area.
Example O:
In the United States, algorithmic decision-making systems have been used for some time by judges to calculate the probability of recidivism of suspected offenders. These systems are intended to generate suggestions for decisions on issues relating to bail or release on probation. This application thus indirectly affects the personal freedom of the person concerned. Moreover, individuals cannot escape such an application because it is applied by the state. Accordingly, there is already a high application-related risk from the outset.
At the same time, studies show that there is a high systemic risk in the form of possible distortions in the results. The software COMPAS (Correctional Offender Management Profiling for Alternative Sanctions), for example, uses 137 parameters to calculate the probability of recidivism. The calculation includes static factors, such as the accused person’s education, their neighbourhood or the length of the criminal record. However, researchers were able to show in 2016 that the algorithm leads to an increase in inequalities. For people of colour, the system incorrectly assumed too high a probability of reoffending. In this way, existing inequalities are reinforced by the use of ADS technology. Another group of researchers has proposed a way of mitigating this problem. This group succeeded in reducing distortion and improving comprehensibility by reducing the number of parameters used. Following this strategy could theoretically reduce the systemic risks of software such as COMPAS to such an extent that the application could be moved from the red to the yellow area (from O to O'). The example thus shows how the matrix not only facilitates the classification of a technology as green, yellow or red, but also draws attention to practical measures that could be taken to reduce the risk.