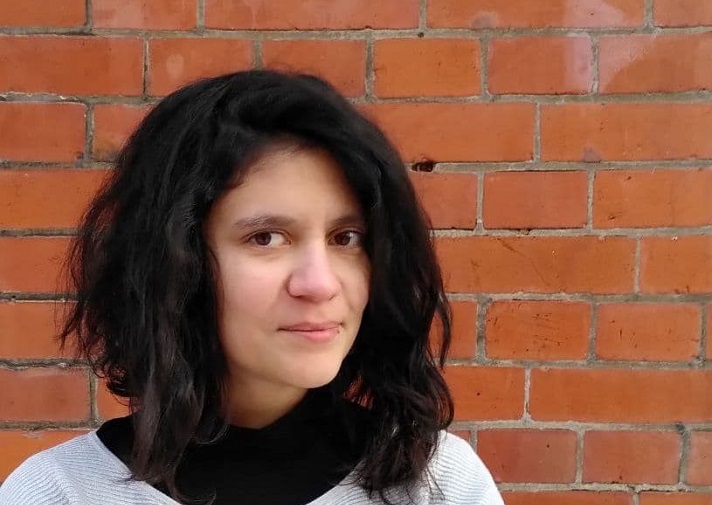
Paola Lopez - Addressing the power struggles of combating algorithmic bias
17.05.2023Algorithmic bias has received a lot of attention over the years, by media as well as scholars. We talked to bias researcher Paola Lopez about what progress has been made, what challenges and power struggles remain.
It’s been 5 years since Joy Buolamwini's and Timnit Gebru's paper “Gender Shades” was published, which brought algorithmic bias to widespread attention and public scrutiny. How much progress have we made in combatting it?
Bias in algorithmic systems has been a topic of scholarly inquiry even longer – Batya Friedman and Helen Nissenbaum published a typology of biases in computer systems as early as 1996. In the last couple of years, the discourse around algorithmic bias has become somewhat of a point of reference that most people can agree on: Bias in algorithmic systems is bad, and we don’t want it. Under the umbrella term bias, many harmful technologies have been analyzed, challenged, critiqued and some of them have even been banished. As most bias tests are quantitative, their results are somewhat accepted by the technical community: Quantitative results are the language of the technical community. Thus, I believe that the bias discourse is a more effective vehicle for concrete and specific change than, say, the ethics discourse. This is, however, also the pitfall of the bias discourse: Bias, when defined robustly, is a lens through which one can analyze one specific algorithmic tool. Underlying questions of societal inequalities, imbalances of resources and power, and capitalist market logics remain unaddressed.
In what shapes and forms does algorithmic bias appear on platforms, and what are the potential harms?
Bias as a concept can be defined for every automated system that has something to do with humans – every system that either processes data that relates to humans, or a system that has a direct effect on humans. There can be biases in recommender systems that decide which content will be shown to users. Within image-based platforms such as Instagram, certain kinds of images might be preferred to others. This entails biases with regard to representation: Which kinds of bodies are made (in)visible? Platforms, especially social media platforms, have become sites in which a big part of our social life takes place. Hence, issues of erasure and invisibilization can be severe. One example that I studied is a tool that was used by Twitter to automatically generate image crops: When a user uploads one or more images to be embedded in a Twitter post, the platform creates a cropped preview that is visible in the timeline. In 2020, some Twitter users raised accusations that Black people were systematically cropped out of the image previews and examples suggested that the tool carried a racial bias.
Where does this bias stem from? What is it a product of?
The image cropping tool in this example was trained to predict the “saliency” of the uploaded images, or rather its “most salient” area. An area is “salient” if humans are expected to look at this area first when they see the image: it is supposed to be the most important and most interesting area of an image, which, of course, is difficult to robustly define, as different people will regard different areas as interesting or important. Then, around this most salient area, a crop would be made to be shown in the preview. So, the question is – on which kind of training data was this saliency prediction trained? This is always one of the most important issues when it comes to bias in machine learning systems. And in this case, the training data consisted of eye-tracking data from individuals: In a standardized test setting, test participants would look at images, while their eye movements were tracked and recorded. So, the saliency-based cropping tool was trained to “imitate” the viewing patterns of the test observers. This is one way that biases can enter such a system: It augments the viewing habits of a few test participants and incorporates these into its cropping decisions. However, I must say that it is, in fact, not clear that the saliency prediction tool really does what it is supposed to do – machine learning tools are, up to a certain degree, opaque in their functionality.
How can systems be tested for bias? What are the pitfalls, what are the difficulties?
One difficulty is that “bias” is such a broad term – however, when one plans to test a specific algorithmic system for bias, one has to precisely define what one means by bias in this case. Quantitative methods require quantification of concepts. And defining what one means by a “biased algorithmic decision” in a way that is methodically robust is difficult. One can either analyze the underlying training data, or curate a specific dataset that is used for testing the behavior of the algorithmic system in question. Both testing approaches rely on decisions by those who test – decisions that are not neutral and themselves entrenched in values and opinions. Thus, there is no “objective” bias test, just as there is no “objective” algorithmic system.
How have platforms responded to algorithmic bias, what are they doing to prevent it?
In the described Twitter case, the platform took the accusations seriously: Researchers of Twitter’s then-ethics team META conducted systematic quantitative bias analyzes of the cropping tool, and they concluded that the tool was, indeed, biased. Then, Twitter removed the machine learning-based cropping modality and changed it to a user agency-based approach: The users themselves, when uploading an image, decide on the placement of the crop, and in most cases, the image would just remain uncropped. Then, in a quite novel way of reacting to accusations, Twitter hosted an “algorithmic bias bounty challenge” in which they invited everyone to find biases and potential harms. They then awarded a few monetary prizes for the best submissions.
What are the problems behind such algorithmic bias bounty hunts?
In this case, what I criticize is the low amount in prize money. Usually, these kinds of bounty hunts are conducted to find IT security vulnerabilities or other bugs in software systems, and the awards amount to much more than the adapted “bias bounty” format hosted by Twitter. In total, Twitter awarded $ 7,000 in bounties, which, for a company like Twitter, is quite low. This created a constellation in which Twitter received lots of submissions by people all over the world who did their work without any monetary compensation – everyone who did not get one of the five prizes received nothing. But at least something happened, and the Twitter ethics team surely did their best to improve the situation within their means and the surrounding corporate logic. Obviously, since then, a lot happened. The ethics team has been disassembled, and when Elon Musk became Twitter’s CEO, the self-representation of Twitter has drastically changed. Ethics and unbiased-ness do not seem to be a concern anymore. The bias bounty format, however, has been and is being adapted, and it might be a good way to address biases in algorithmic systems in the future – as long the participants get compensated adequately for their work.
What has resistance to biased algorithms looked like in the past, and how do you think it could evolve?
Studies on bias, NGOs, academic scholarship, activists, journalistic articles, science communication, individual users who post, re-post, and comment on bias, reports by international organizations – they form a “swarm of points of resistance”, as Michel Foucault would say. It is a collective effort that is not at all homogeneous, but in itself contains struggles and conflicts. What does “bias” mean? How does bias as a concept fall short in addressing social injustice? How can we productively engage with the inherent limitations of bias testing? That there are all these open questions and conflicts is, I think, a fundamentally good thing. After all, they concern the question of how we want to live and communicate.
Thank you for the interview!
Paola Lopez is an Associate Researcher at the Weizenbaum Institute research group Technology, Power and Domination. A mathematician by training, she is currently working at the Department of Legal Philosophy at the University of Vienna. She examines questions of (in)justice that emerge from the deployment of data-based algorithmic systems, and has developed a socio-technical typology of biases. She wrote the first published analysis of the Austrian AMS algorithm and its potential for discriminatory effects, and examined the bias discourse around Twitter’s saliency-based image cropping algorithm. Lopez was awarded as one of ten AI Newcomers of 2023 by the German Informatics Society and the German Federal Ministry of Education and Research.
She was interviewed by Leonie Dorn