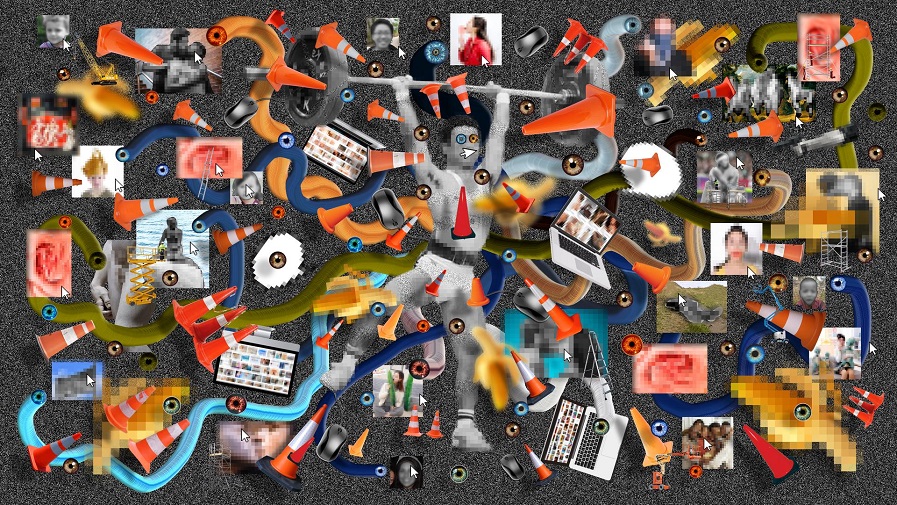
Data workers - The working conditions and importance of the people behind AI
04/29/2024From precarious conditions to gender-specific challenges, those are the experiences that the sociologist and computer scientist Milagros Miceli has encountered in her research. We talked with her about the world of data workers, and also about how their work influences technologies and society.
How did you get into this field of research, what made you want to investigate data work?
I am a sociologist and a computer scientist, but six years ago, when I started at the Weizenbaum Institute in a research group that, up until that point, only included computer scientists, I was just a sociologist. I'm saying “just” because when you enter certain spaces as a social scientist, you’re often looked at – at first – as “okay well, but can you really bring something to the equation, if you're not acquainted with the technical details. Can you really speak about this issue?” I had never worked in tech, had never done anything related to tech. But the colleagues in the research group had much more faith in what I could bring to the table than myself. They were all working on really cool projects, on harms produced by AI and ethics of AI but focusing on bias mitigation tools and explainability techniques. But I needed to look at these issues through the lens of the social relationships that come with them. I needed to know who are the humans behind AI. And I started looking at data scientist first, and then I realized there was an area that was completely ignored by many in the field, and that was the field of data work and data workers.
Now you’re a sociologist and a computer scientist. How do these disciplines each tackle the field of data work, and how has it helped you in your research that you can combine these two?
I think, even though my PhD is in computer science, I still remain first and foremost a sociologist and that has to do with the methods that I use and also the questions that I ask. Those have to do with social relationships, social hierarchies, power dynamics. And I think more and more computer scientists and researchers in general are realizing the value in that.
When I started six years ago, that was not the mainstream. Typically, you would have labor sociologists talking about wages and labor conditions in data work, but completely detached from the consequences that those had on the computer systems or on the data. And then, you would have the computer scientists talking about data work – data annotation, for example and about biases. And they would be starting from the point that humans are biased, and that we therefore need to tame and contain and restrain these workers and their subjectivities. And those were the two strains, but they wouldn’t be talking to each other. And especially the question of workers subjectivities would lead to more precarization of the workers. Because if you consider data workers to be bias carriers more or less, and a hazard to the data, you would surveil and restrain them even more, which is what commonly happens in data work.
So that's where my work comes in, arguing, that one thing has to do with the other. On the one hand, if you gave workers more space for deliberation, or to talk to each other, you would be able to create better data, which would actually be beneficial for the systems. And on the other hand, instead of aiming at debiasing, there’s a long tradition in the social sciences, that says that all data is biased. There is no data that is not biased. The questions is how we deal with that.
How long has this industry of data work existed, how much has it grown and is expected to grow?
Data work has existed forever. The question is the professionalization of the data workers and also when it started existing at a scale. That we can track back to the first data work platform or data annotation platform, Amazon Mechanical Turk, that exists since 2005. So almost 20 years. And it’s interesting to observe the trajectory of the developments in the space of AI since MTurk started, what has been enabled by the mere existence of a workforce that suddenly was available at low prices, in a large scale, at 24 /7. So, there is a correlation between the ability to hire these workers at scale for cheap prices and the ability to produce the systems that we know today.
It is very difficult to know exactly how many data workers there are. But there is a report from the World Bank published last year that estimates that there are between 150 and 430 million data workers worldwide, and that the number has grown exponentially in the past decade. The actual number is probably not too far off.
This also contradicts those comments predicting that we won't need data workers in the future. The tasks may change, but the need for them is still there, and it grows.
What are the working conditions that you've encountered in your research?
Well, the conditions can be summarized as being bad. The main problem is in the outsourcing itself, because nobody feels responsible if things go south or if something happens to the workers. In the famous example of OpenAI outsourcing data work through the company Sama, in Kenya, the workers were being confronted with material that was detrimental to their mental health and in many cases, they were disabled by the job. We are working with five of these workers at the moment, and they have told us that they have so much post-traumatic stress disorder (PTSD) that they couldn't go back to doing that job, or get other jobs. When they demanded compensation, Sama would say, "Well, the material didn't come from us. It was OpenAI." And OpenAI said, "Well, we don't know those workers. We didn't hire them. We hired Sama."
Another problem has to do with the fact that many of these workers work on platforms like MTurk, Prolific, and Upwork, which means they only get paid per task, not for the time that it takes them to actually complete the job. And they are only paid a few cents per task. That opens up room for price discrimination. A worker here in Germany would be paid differently for the same task than someone in Venezuela, for example.
Another practice often happening on platforms are mass rejections. That means that clients can put a task on the platform which is then completed by the worker, but when the clients are in some way unhappy with the data, they can choose not to pay them. But they still get to keep the data that was produced. And the reasons for this are completely arbitrary.
All this makes it very difficult for data workers to know how much they will be paid at the end of the week or month, whether they will be able to pay rent, for example. Some people have tried to portray this work as something that workers do for fun, or as an extra income, or to buy themselves nice things. There might be such cases, but that's not the reality of millions of data workers worldwide.
You've already mentioned price discrimination, but are there other ways how working conditions vary between global north and global south?
They vary in terms of the protections that workers have. In some countries, workers are more protected than in others, or there are things that platforms can or cannot do.
Then there are other, more subtle things, such as the time it takes workers to understand a task or navigate a platform in a language they oftentimes don't speak. Many of the platforms operate with tasks that are only posted in English. The instructions for a task are sometimes only one page long, but I've also seen instructions with up to 90 pages. The best paid tasks usually have longer instructions. Workers are not paid for the time it takes them to translate and make sense of such tasks, so this is another form of wage theft.
What about gendered differences to the work?
Typically, the overall conditions are the same. However, due to the gendered vulnerability of individuals, this job can be more harmful for non-male, and also for non-cisgender people. I’m referring here to issues that go beyond the purely economic.
In many cases, you're going to be reviewing material that is considered sensitive, like images of violence, hate speech, sexual violence. If you are a transgender person, a woman, or a non-binary person, this may affect you much more because chances are that you have experienced this kind of violence yourself. This is why many of the female and non-binary workers I have interviewed rely on Facebook groups or WhatsApp groups to warn each other about certain tasks. Because many of these tasks don't even come with a trigger warning, so you only find out if the images or data are bad for your mental health once you've already started. It is worth mentioning here that most platforms explicitly warn workers about discussing the tasks with other workers. But, even if this is not allowed by the platforms, many data workers rely on groups and forums as a form of self-protection.
What relationship to technology do the workers describe to you? Does their work have an effect on how they use technology, like social media or other platforms?
That has evolved. In the first interviews I conducted in 2018, I asked about something that had the word "machine learning" in it, and many workers didn’t know what it was. “AI” was not the first thing that came to mind when they described their work.
Right now, it’s different. Workers tell us that their kids are not allowed to have social media or use ChatGPT, and that they themselves don't have social media or cover the camera on their laptops. Awareness of how dangerous and extractive these industries are and how our data has been taken from the most uncommon places has really grown. One year ago, data workers from Venezuela even anonymously tipped off the press about how they had been labeling images of people in their homes that had been taken by robot vacuum cleaners. So they are also warning us.
How do you go about your research? What are the challenges?
I go to the places where the workers are, I try to immerse myself, try to do the work even, talk to people and work with them. I refuse to research, write, and speak about data workers from the comfort of my desk. I don’t do research on data workers but try to do research with them. I don't want to be another one of those people who just extracts data from them.
In this spirit, we have been working on a project that is called “The Data Workers Inquiry”, in which data workers in different places of the world are our co-researchers. This is what some have called community-based research. The data workers center their own research questions and control the narrative. They report on their work and experiences from their own perspective and in their own terms. We are engaging 15 data workers in different regions, who investigate various aspects of their work. We have people who look into drug abuse among their co-workers because of the psychological consequences, others are looking into gender perspectives or migration. Another co-researcher is looking at the communication between data workers and clients, how important it is and how beneficial for the data. The presentation of these findings is also according to the co-researchers’ preferences: we’ll have a zine, podcasts, a video documentary, an animation film, reports, essays, pictures.
The challenges with this work change over time. In the beginning of course it was reaching the workers. Right now, the challenge is to remain healthy through this all. It is very emotionally taxing to be working on this and to be limited in what you can do about it.
It was also not easy to get funding for The Data Inquiry Project, to pay data workers the hourly rate that we as researchers get. I really want them to own the products they create and to be paid accordingly. Because at the end of the day, research is a job, nobody does this just for the fun of it.
We’ve had this hype on AI for over a year now. Has that helped your research and the experiences of data workers to become more visible? What has annoyed you about this discourse on AI?
If the hype has caused for the press to be interested in my work, I think it has to do with the press always looking for a counterpart to the hype. So that's the space in which I'm generally called upon – to answer to the BS. I prefer to discuss my research from its own perspective rather than in opposition to something else. But I do critical research, indeed. So this part of the job.
I take all the opportunities I can get to speak with the press, and make these issues visible. I want this to be on the news. But I also reflect on why it’s me that gets to sit in front of the camera and not the data workers. They of course are often subjected to NDAs and cannot speak freely. When they do, it’s great for the numbers and ratings, but if the workers face trouble or retaliation, nobody reacts or intervenes. I think the Data Workers’ Inquiry comes to fill this gap: it is a repository of data workers’ accounts, unfiltered. But it is also done on workers’ terms, not following the urgencies of the press or chasing academic KPIs.
Do you think that this media attention will help to improve the working conditions? And if not, what will?
I think visibility is a great thing, but it is not nearly enough. What comes now is pressure on the politicians, pressure on the companies. Pressure to create regulations that keep the well-being of the workers, the employees as well as the platform workers, in mind.
What doesn't give me a lot of hope are these fake crocodile tears of Elon Musk and Sam Altman and the whole bunch. They’re crying about the existential risks of AI, but then block every attempt of effective and independent regulation, and for them to actually be held accountable. And it doesn’t give me hope that most people in power take them seriously.
We also need the general public to be conscious that this is not just about random workers somewhere in another continent, very far away from us. These workers are decisive in creating the technologies that we all use and that will be judging us, that will decide on our access to resources, or identifying us. So taking care of it means taking care of all of us, including our families, our kids and ourselves. I don't think this has become clear enough when we talk about it. We also need to be more aware about who the companies are that support unjust or potentially harmful AI systems, and then boycott them.
What gives you hope?
What gives me hope is the younger generation of students who are more and more interested in this space. The people from computer science that want to be more than just technologists and see the value in collaborating with other disciplines, like social science.
I’m also hopeful about spaces outside of academia and big tech, like NGOs, for example, where these issues are thought of outside of techno-capitalism, but also not tied to the typical performance metrics that in many cases constrain academia.
Fortunately, there is a growing tendency to see technologies as tools that shouldn’t control us, but that we can create and use for the benefit of our communities. And I love to see a variety of voices speaking up that otherwise doesn’t get heard – from different geographical regions, from indigenous spaces, queer spaces, from workers’ unions and advocacy organizations. There is a knowledge wealth in these spaces that doesn’t make it to mainstream media but is so incredibly important for the future. People in these spaces are subverting the status quo and I am very grateful for that.
What will you be working on next?
The Data Workers Inquiry will be launching very soon, on July 8th, with an event where we will present the investigations that the data workers have produced.
We will also keep working on the connection between better labor conditions and better data. So, we are trying to measure the performance of specific data sets and checking what the variations are that cause those data sets and those models to perform better. If you pay workers more, will the model perform better? If you give workers instructions in their own language, does that affect the data they produce? Do workers who are employed by the company generate better data than people who work for platforms? We want to produce numbers in order to be able to influence first researchers, but then also industry practitioners. Because not many engineers or AI companies read or trust qualitative research, they read numbers.
We are also working on a set of guidelines for academic requesters looking to outsource data work. We’re thinking of submitting these guidelines to associations like the DFG (Deutsche Forschungsgemeinschaft - German Research Foundation) but also to the ACM. That's the Association for Computing Machinery in the US, the largest association for computer science. So, we are trying to have those included in the ethics codes of the respective associations, and specific institutions’ ethics boards, like the Weizenbaum Institute, for example. Here we are trying to collaborate with the new ethics board committee to see if it is possible to include something similar in their guidelines. In the same way in which we have guidelines on how we treat interview participants or generally research participants, we should have guidelines in terms of how we treat data workers that work for us in advancing research.
Thank you for the interview!
Dr. Milagros Miceli leads the research Group “Data, Algorithmic Systems and Ethics“ at the Weizenbaum Institute. Her research is centered on exploring the production of ground-truth data for machine learning, with a specific focus on labor conditions and power dynamics involved in data generation and labeling. Dr. Miceli is interested in analyzing the underlying questions of meaning-making, knowledge production, and symbolic power embedded within machine learning data. Dr. Miceli's work sheds light on the ethical and social implications of AI development, especially data work.
She was interviewed by Leonie Dorn
artificial&intelligent? is a series of interviews and articles on the latest applications of generative language models and image generators. Researchers at the Weizenbaum Institute discuss the societal impacts of these tools, add current studies and research findings to the debate, and contextualize widely discussed fears and expectations. In the spirit of Joseph Weizenbaum, the concept of "Artificial Intelligence," is also called into question, unraveling the supposed omnipotence and authority of these systems. The AI pioneer and critic, who developed one of the first chatbots, is the namesake of out Institute.