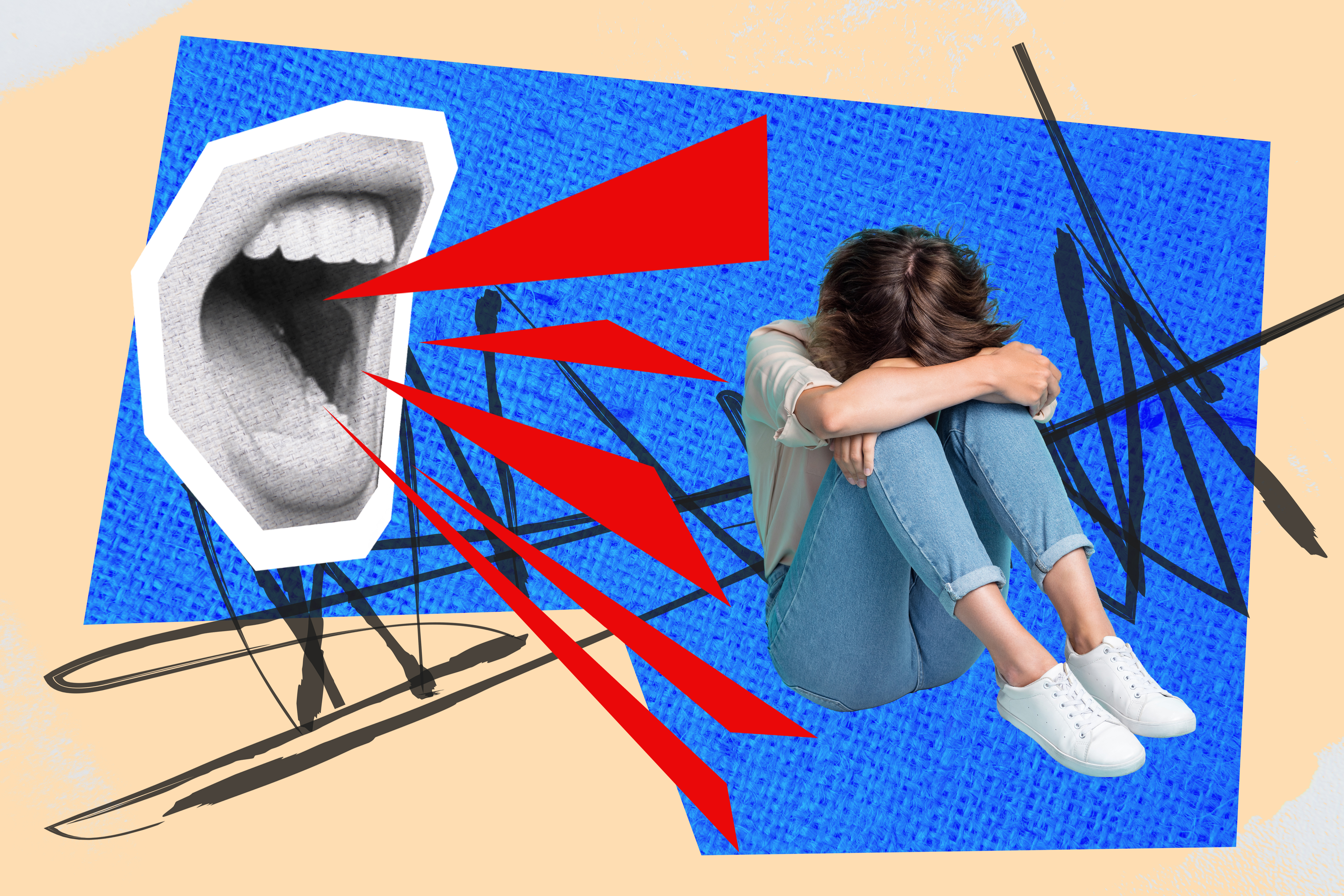
Lost in Content Moderation
04/14/2025Do commercial content moderation APIs over- or under-moderate group-targeted hate speech? Weizenbaum researcher David Hartmann takes on this question in a new paper that has earned him a spot at the renowned CHI 2025 Conference on Human Factors in Computing Systems. We spoke to him about his research.
Recently your paper “Lost in Moderation: How Commercial Content Moderation APIs Over- and Under-Moderate Group-Targeted Hate Speech and Linguistic Variations” was accepted to the CHI 2025 Conference on Human Factors in Computing Systems – the premier international gathering focused on Human-Computer Interaction. Congratulations! Can you tell us more about your research?
David Hartmann: Yes, I’m really looking forward to presenting our work at CHI 2025 in Yokohama! The project is a joint effort by the Weizenbaum Institute, Hertie School Berlin, the Center for Advanced Internet Studies in Bochum, the University of Wuppertal, and Technische Universität Berlin.
We were curious about how well AI-based content moderation works, especially since there are now several commercial tools that offer moderation “as a service” via APIs, something that smaller blogs, newspapers, or website owners can easily use. These systems therefore moderate content of millions to billions of people and until now a systematic evaluation of performance and potential systematic disparities and failures is missing.
This was particularly concerning for us as these systems pose a certain risk. When harmful content isn’t moderated — what we call under-moderation —users are left exposed to hate speech, particularly those from marginalized communities. On the other hand, when legitimate content is wrongly taken down — over-moderation — it limits people’s ability to express themselves and participate in public discourse. This tension is what’s often referred to as a “wicked problem”: there’s no perfect solution everyone will agree on. This is a serious issue.
The use of AI in moderation introduces additional risks, as over- and under-moderation can scale quickly and often without enough transparency or oversight. These risks are particularly concerning for groups defined by protected characteristics such as gender, race, or religion.
In our study, we evaluated the five largest providers, including OpenAI and Amazon, by conducting a full-scale audit with over 5 million queries. The project took us more than a year! Our findings corroborate prior research on the complexities of detecting non-explicit hate speech and counter-speech with AI, highlighting the need for continued recalibration and improvement. For example, we found that the APIs often rely on identity terms like “Black” to predict hate speech. While services by OpenAI and Amazon perform slightly better, all providers show significant weaknesses when it comes to moderating implicit hate speech — such as coded or subtle language and irony — especially when directed at LGBTQIA+ individuals. They also struggle with counter-speech, reclaimed slurs, and content related to Black, LGBTQIA+, Jewish, and Muslim communities.
Why is it important to conduct research on content moderation techniques and hate speech?
I previously mentioned that these systems impact content created by millions to billions of users. Additionally, content moderation on social media – especially when involving AI – is often opaque, with limited understanding of how AI contributes to the process. We believe that society needs oversight over socio-technical systems that make real-world decisions, such as determining what content gets deleted online and what remains accessible.
Content moderation on social media – especially when involving AI – is often opaque, with limited understanding of how AI contributes to the process.
Algorithmic content moderation is employed to address online hate speech, but it is not infallible. Content moderation can systematically fail in two ways: (1) over-moderation and (2) under-moderation of specific groups or linguistic variations. The issue with AI is that, because this process is automated and used across multiple platforms, failures in moderation can scale across the internet. Research has shown that systematic under-moderation of hate speech can decrease users' sense of safety, reduce participation from affected groups, and reinforce harmful stereotypes. Ultimately, this can lead to real-world harm and violence. On the other hand, systematic over-moderation of marginalized voices can lead to self-censorship and exclusion from online platforms. If content moderation algorithms consistently flag counter-speech and reclaimed language as offensive, users from marginalized communities may feel discouraged from participating in online discussions.
As I mentioned earlier, we weren’t surprised to find biases in these systems. Similar problems are already known from non-commercial models that researchers or users run on local machines. But it’s important to ask whether commercial providers, who offer these services at scale, have effectively addressed these known issues — or whether the same shortcomings persist.
What perspectives can your study contribute to the current scientific debate?
I think there are three main contributions our research makes.
First, our study highlights disparities in how well these systems perform for different sender and target groups in the context of hate speech. Based on these findings, we recommend that providers recalibrate their moderation models to reduce both over- and under-moderation. Crucially, this should be done in collaboration with marginalized communities and NGOs, ensuring that lived experiences help inform moderation strategies. Companies should test their models specifically for the types of biases we identified, and make sure their tools can moderate content equally well and in a fair manner across all groups.
Second, we provide a reproducible framework for evaluating algorithmic content moderation systems, even without access to the model’s internals (a so-called “black-box” approach). My research focuses on third-party audits; independent evaluations of commercial AI systems. I want to contribute methods that NGOs, journalists, civil society actors, and academics can use. This includes a systematic audit methodology and tools that can be reused in future work.
Third, our findings corroborate calls from other researchers for more transparency and access. It’s concerning that many moderation API services offer very limited information about their underlying models, training data, or fairness assessments. This leaves those deploying the services without enough insight to evaluate them properly. Content moderation providers should offer clearer guidance and more transparency, especially around the limits of their models when dealing with linguistic nuances or implicit hate speech.
What are the next steps in your research?
My next research builds on this audit work in two directions. In one of my upcoming projects, I aim to make algorithmic audits more accessible for researchers, journalists, and civil society groups. These kinds of audits can be expensive, especially when they involve querying commercial content moderation systems, where every interaction can incur a cost. I’m exploring ways to make these investigations more efficient by developing smarter strategies that allow us to draw meaningful conclusions while minimizing resource use.
Another focus of my work is understanding how online platforms moderate content, particularly during sensitive times like elections. So far, it’s been nearly impossible to assess what content gets taken down on major platforms like Facebook, Instagram, or TikTok. But new regulations in the EU, such as the Digital Services Act, are opening up possibilities for more transparency.
With that in mind, we are preparing a project that brings together data analysis, expert interviews, and policy documents to examine patterns of content moderation during election periods. The broader goal is to help build a toolkit for holding AI systems accountable, particularly when their decisions are invisible, automated, and affect public discourse.
Thank you very much!
David Hartmann is a doctoral researcher in the research group “Data, Algorithmic Systems, and Ethics” at the Weizenbaum Institute and at Technische Universität Berlin. He investigates the technical-mathematical and philosophical-ethical aspects of algorithmic fairness and fair machine learning models, with a particular focus on auditing discriminatory algorithms, causal inference, and textual online data.
Interview by Moritz Buchner